-
Notifications
You must be signed in to change notification settings - Fork 131
New issue
Have a question about this project? Sign up for a free GitHub account to open an issue and contact its maintainers and the community.
By clicking “Sign up for GitHub”, you agree to our terms of service and privacy statement. We’ll occasionally send you account related emails.
Already on GitHub? Sign in to your account
Incorrect Solutions with Theseus compared to Ceres. #499
Comments
Hi @Muon2. Thanks for issue. It's worth pointing out that we don't yet support line search, which makes direct comparison with Ceres more difficult. In this case, one possibility is that Theseus is jumping around the local optimum because of the large step size. Two easy things to try to start with are:
Also, can you add Thanks. |
Thanks for your advice. Here is the updated setting: optimizer = th.LevenbergMarquardt(
objective,
linear_solver_cls=th.CholeskyDenseSolver,
linearization_cls=th.DenseLinearization,
linear_solver_kwargs={'check_singular': False},
vectorize=True,
max_iterations=1000,
step_size=0.01,
abs_err_tolerance = 1e-12,
rel_err_tolerance = 1e-10,
)
...
with torch.no_grad():
updated_inputs, info = theseus_optim.forward(theseus_inputs,
optimizer_kwargs={
"track_best_solution": True,
"verbose": True,
'adaptive_damping': True
}) Output:
Although Theseus gets closer to the ground-truth solution, it requires much more iterations compared to Ceres, which achieves exact zero error with only a FEW steps (~5). |
I also encountered the similar problem |
As mentioned above, please keep in mind that we haven't yet added support for line search methods, so we only have basic control over the step sizes taken by the optimizers. It's expected that Ceres will be much more efficient, considering that it provides many sophisticated line search methods that can have huge impact in performance. Also, keep in mind that Theseus is not meant as a replacement for Ceres. Our target applications are models that need differentiable optimization layers as part of larger neural architectures. In such cases, optimization accuracy might be less important, since the parameters of your optimization problem constantly change throughout learning. That being said, if this error is problematic for your application we can consider increasing priority for adding some form of line search. But if you are concerned about potential bugs and just want to validate with Ceres, you could try disabling line search in Ceres (@fantaosha might have some pointers here), and see how we compare. We would definitely be interested in knowing the outcome of this comparison! |
Also, looking at your logs, I'd say using both |
Thank you for your reply. I'm currently trying to use Theseus in neural networks, and optimization accuracy is crucial in my setting because my forward function is sensitive to the input number (it's related to skinning in computer graphics). If Theseus cannot achieve similar accuracy, it will result in unacceptable geometry artifacts. I'm looking forward some form of line search so that I can fully differentiate my pipeline. |
Got it, thanks for clarifying. Did you try any of the suggestions in my last post? Curious if any combination of hyperparameters would suffice for your application. |
@Muon2 did you notice any improvements with other optimization parameters? |
@luisenp, sorry for the late reply. I tried multiple combinations of hyperparameters, but it still requires a lot of time and does not converge to an acceptable precision. I will keep an eye on following updates. |
I also stumbled onto this issue while trying to port the basic example from scipy: I wasn't expecting to have to tuning any hyperparameters to get this to converge. It might be good to add a note on this in the main README until a line search is implemented (I guess related to #153). |
❓ Questions and Help
I'm currently using Ceres with the following options:
While Ceres is giving me exact solutions, I'm encountering issues when using Theseus as it cannot give me correct solutions. Here's the code I'm using with Theseus:
Do you have any ideas on what could be causing this issue? Is it possible that Theseus is not as efficient as Ceres?

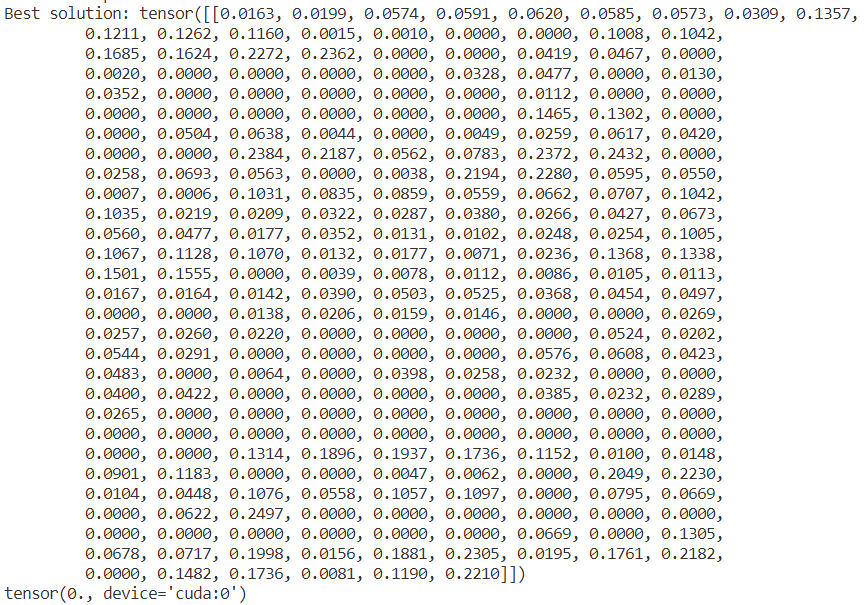
I cannot give specific code, but as it can be seen in following pictures, ceres successfully gives the ground-truth solution of 0.2. I have tried my best to align the optimizer hyperparameters (such as iterations or numerical precisions), but I'm still not able to get the correct solution (only approximated) from Theseus.
Ceres's solution:
Theseus's solution:
The text was updated successfully, but these errors were encountered: